Change management professionals are increasingly requested to provide measurement, data, and insights to various stakeholder groups. Not only does this include tracking various change outcomes such as business readiness or adoption, but stakeholder concerns also include such as change saturation and visibility of incoming initiative impacts.
To become better at working with data there is much that change managers can learn from data scientists (without becoming one of course). Let’s explore how change management can benefit from the practices and methodologies employed by data scientists, focusing on time allocation, digital tools, system building, hypothesis-led approaches, and the growing need for data and analytical capabilities.
1. Time Allocation: Prioritizing Data Collection and Cleansing
Data scientists spend a substantial portion of their time on data collection and cleansing. According to industry estimates, about 60-80% of a data scientist’s time is dedicated to these tasks. This meticulous process ensures that the data used for analysis is accurate, complete, and reliable.
In the below diagram from researchgate.net you can see that for data scientists the vast majority of the time is spent on collecting, cleansing and organising data.
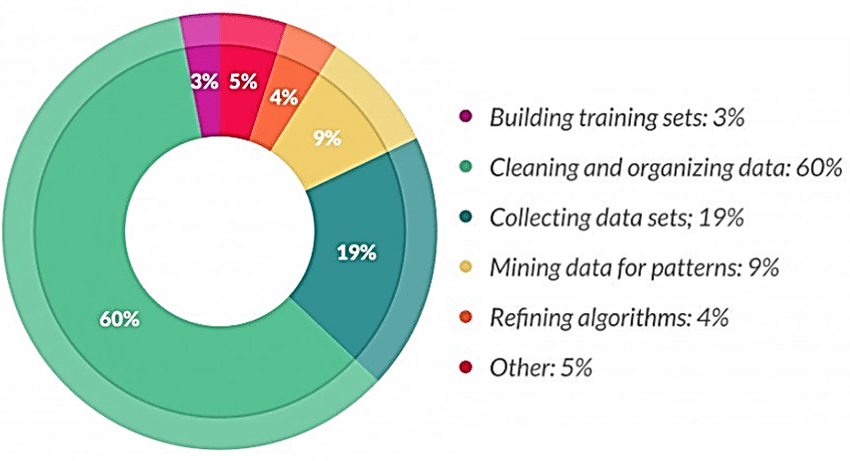
You might say that change managers are not data scientists because the work nature is different, and therefore should not need to carve out time for these activities? Well, it turns out that the type of activities and proportions of time spent is similar across a range of data professionals, including business analysts.
Below is the survey results published by Business Broadway, showing that even business analysts and data analysts spend significant time in data collection, cleansing, and preparation.
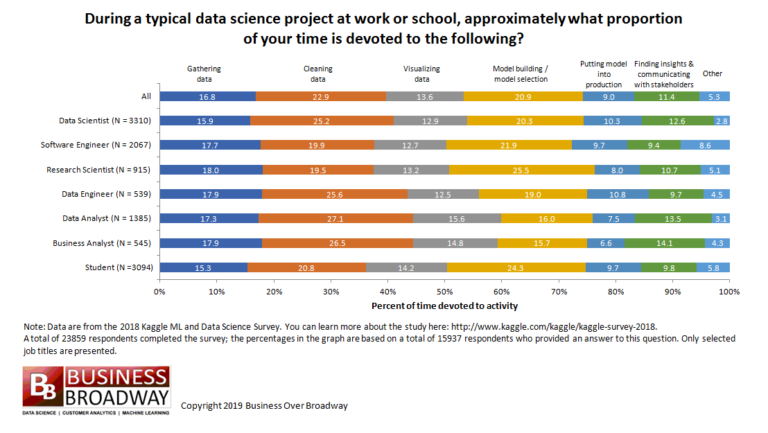
Lessons for Change Management
a. Emphasize Data Collection and Cleansing: For change managers, this translates to prioritizing the collection of reliable data related to change initiatives. This might include stakeholder feedback, performance metrics, impact data and other relevant data points. Clean data is essential for accurate analysis and insightful decision-making. Data projects undertaken by change managers are not going to be as large or as complex as data scientists, however the key takeaway is that this part of the work is critical and sufficient time should be allocated and not skipped.
b. Allocate Time Wisely: Just as data scientists allocate significant time to data preparation, change managers should also dedicate sufficient time to gathering and cleaning data before diving into analysis. This ensures that the insights derived are based on accurate and reliable information.
It also depends on the data topic and your audience. If you are presenting comparative data, for example, change volume across different business units. You may be able to do spot checks on the data and not verify every data line. However, if you are presenting to operations business units like call centres where they are very sensitive to time and capacity challenges, you may need to go quite granular in terms of exactly what the time impost is across initiatives.
c. Training and Awareness: Ensuring that the change management team understands the importance of data quality and is trained in basic data cleansing techniques can go a long way in improving the overall effectiveness of change initiatives. Think of scheduling regular data sessions/workshops to review and present data observations and findings to enhance the team’s ability to capture accurate data as well as the ability to interpret and apply insights. The more capable the team is in understanding data, the more value they can add to their stakeholders leveraging data insights.
2. Leveraging Digital Tools: Enhancing Efficiency and Accuracy
Data scientists rely on a variety of digital tools to streamline their work. These tools assist in data collection, auditing, visualization, and insight generation. AI and machine learning technologies are increasingly being used to automate and enhance these processes.
Data scientists rely on various programming, machine learning and data visualisation such as SQL, Python, Jupyter, R as well as various charting tools.
Lessons for Change Management
a. Adopt Digital Tools: Change managers should leverage digital tools to support each phase of their data work. There are plenty of digital tools out there for various tasks such as surveys, data analysis and reporting tools.
For example, Change Compass has built-in data analysis, data interpretation, data audit, AI and other tools to help streamline and reduce manual efforts across various data work steps. However, once again even with automation and AI the work of data checking and cleansing does not go away. It becomes even more important.
b. Utilize AI and Machine Learning: AI can play a crucial role in automating repetitive tasks, identifying patterns, data outliers, and generating insights. For example, AI-driven analytics tools can help predict potential change saturation, level of employee adoption or identify areas needing additional support during various phases of change initiatives.
With Change Compass for example, AI may be leverage to summarise data, call out key risks, generate data, and forecast future trends.
c. Continuous Learning: Continuous learning is essential for ensuring that change management teams stay adept at handling data and generating valuable insights. With greater stakeholder expectations and demands, regular training sessions on the latest data management practices and techniques can be helpful. These sessions can cover a wide range of topics, including data collection methodologies, data cleansing techniques, data visualisation techniques and the use of AI and machine learning for predictive analytics. By fostering a culture of continuous learning, organizations can ensure that their change management teams remain proficient in leveraging data for driving effective change.
In addition to formal training, creating opportunities for hands-on experience with real-world data can significantly enhance the learning process. For instance, change teams can work on pilot projects where they apply new data analysis techniques to solve specific challenges within the organization. Regular knowledge-sharing sessions, where team members present case studies and share insights from their experiences, can also promote collective learning and continuous improvement.
Furthermore, fostering collaboration between change managers and data scientists or data analysts can provide invaluable mentorship and cross-functional learning opportunities. By investing in continuous learning and development, organizations can build a change management function that is not only skilled in data management but also adept at generating actionable insights that drive successful change initiatives.
3. Building the Right System: Ensuring Sustainable Insight Generation
It is not just about individuals or teams working on data. A robust system is vital for ongoing insight generation. This involves creating processes for data collection, auditing, cleansing, and establishing governance bodies to manage and report on data.
Governance structures play a vital role in managing and reporting data. Establishing governance bodies ensures that there is accountability and oversight in data management practices. These bodies can develop and enforce data policies, and oversee data quality initiatives. They can also be responsible for supporting the management of a central data repository where all relevant data is stored and managed.
Lessons for Change Management
a. Establish Clear Processes: Develop and document processes for collecting and managing data related to change initiatives. This ensures consistency and reliability in data handling.
b. Implement Governance Structures: Set up governance bodies to oversee data management practices. This includes ensuring compliance with data privacy regulations and maintaining data integrity. The governance can sponsor the investment and usage of the change data platform. This repository should be accessible to stakeholders involved in the change management process, promoting transparency and collaboration. Note that a governance group can simply be a leadership team regular team meeting and does not need to be necessarily creating a special committee.
c. Invest in system Infrastructure: Build the necessary system infrastructure to support data management and analysis that is easy to use and provides the features to support insight generation and application for the change team.
4. Hypothesis-Led Approaches: Moving Beyond Descriptive Analytics
Data scientists often use a hypothesis-led approach, where they test, reject, or confirm hypotheses using data. This method goes beyond simply reporting what the data shows to understanding the underlying causes and implications.
Lessons for Change Management
a. Define Hypotheses: Before analyzing data, clearly define the hypotheses you want to test. For instance, if there is a hypothesis that there is a risk of too much change in Department A, specify the data needed to test this hypothesis.
b. Use Data to Confirm or Reject Hypotheses: Collect and analyze data to confirm or reject your hypotheses. This approach helps in making informed decisions rather than relying on assumptions or certain stakeholder opinions.
c. Focus on Actionable Insights: Hypothesis-led analysis often leads to more actionable insights. It is also easier to use this approach to dispel any myths of false perceptions.
For example: Resolving Lack of Adoption
Hypothesis: The lack of adoption of a new software tool in the organization is due to insufficient coaching and support for employees.
Data Collection:
- Gather data on the presence of managerial coaching and perceived quality. Also gather data on post go live user support.
- Collect feedback from employees through surveys regarding the adequacy and clarity of coaching and support.
- Analyse usage data of the new software to identify adoption rates across different departments.
Analysis:
- Compare adoption rates between employees who received sufficient coaching and support versus those who did not.
- Correlate feedback scores on training effectiveness with usage data to see if those who found the training useful are more likely to adopt the tool.
- Segment data by department to identify if certain teams have lower adoption rates and investigate their specific training experiences.
Actionable Insights:
- If data shows a positive correlation between coaching and support, and software adoption, this supports the hypothesis that enhancing coaching and support programs can improve adoption rates.
- If certain departments show lower adoption despite completing coaching sessions, investigate further into department-specific issues such as workload or differing processes that may affect adoption.
- Implement targeted interventions such as additional training sessions, one-on-one support, or improved training materials for departments with low adoption rates.
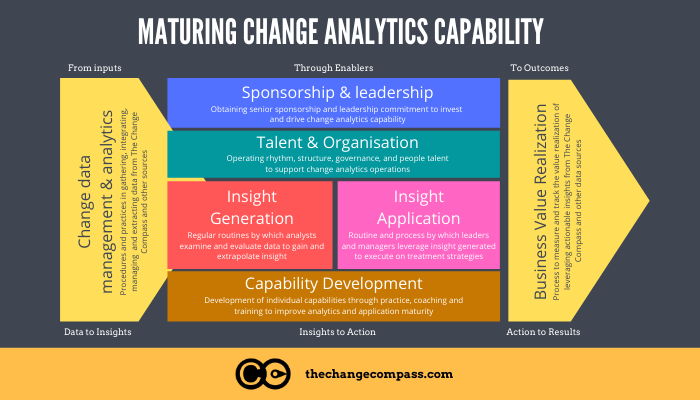
5. Building Data and Analytical Capabilities: A Core Need for Change Management
As data and analytical capabilities become increasingly crucial, change management functions must build the necessary people and process capabilities to leverage data-based insights effectively.
Lessons for Change Management
a. Invest in Training: Equip change management teams with the skills needed to manage data and generate insights. This includes training in data analysis, visualization, and interpretation.
b. Foster a Data-Driven Culture: A lot of organisations are already on the bandwagon of Encourage a culture where data is valued and used for decision-making. The change management needs to promote this equally within the change management function. This involves promoting the use of data in everyday tasks and ensuring that all team members understand its importance. Think of incorporating data-led discussions into routine meeting meetings.
c. Develop Analytical Frameworks: Create frameworks and methodologies for analyzing change management data. This includes defining common key metrics, setting benchmarks, and establishing protocols for data collection and analysis for change data. Data and visual templates may be easier to follow for those with lower capabilities in data analytics.
Practical Steps to Implement Data-Driven Change Management
To integrate these lessons effectively, senior change practitioners can follow these practical steps:
- Develop a Data Strategy: Create a comprehensive data strategy that outlines the processes, tools, and governance structures needed to manage change management data effectively.
- Conduct a Data Audit: Begin by auditing the existing data related to change management. Identify gaps and areas for improvement.
- Adopt a Hypothesis-Led Approach: Encourage the use of hypothesis-led approaches to move beyond descriptive analytics and derive more meaningful insights.
- Invest in Technology: Invest in the necessary digital tools and technologies to support data collection, cleansing, visualization, and analysis.
- Train the Team: Provide training and development opportunities for the change management team to build their data and analytical capabilities.
- Collaborate Across Functions: Foster collaboration between change management and data science teams to leverage their expertise and insights.
- Implement Governance Structures: Establish governance bodies to oversee data management practices and ensure compliance with regulations and standards.
By learning from the practices and methodologies of data scientists, change management functions can significantly enhance their effectiveness. Prioritizing data collection and cleansing, leveraging digital tools, building robust systems, adopting hypothesis-led approaches, and developing data and analytical capabilities are key strategies that change management teams can implement. By doing so, they can ensure that their change initiatives are data-driven, insightful, and impactful, ultimately leading to better business outcomes.
To read more about change analytics and change measurement check out our other articles.
To read more about maturing change management analytics check out our infographic here.